AI and Data Privacy: Securing Data in Machine Learning Era
Artificial intelligence and machine learning are the wonder tools in the modern digital ecosystem-promised to bring so many breakthroughs but also as well threats to privacy. AI algorithms might allow for real-time, highly personalized experiences or optimize business processes-even contribute to scientific breakthroughs-but such potential is all predicated on massive amounts of user data. Today, in full swing, data privacy becomes a very critical issue as sensitive information needs to be protected against misuse and unauthorized access besides ethical mishandling.
We then look at how AI and data privacy may intersect, including security challenges to machine learning, and then strategies to balance security with innovation without reducing that.
Importance of Data Privacy in AI:
Data privacy in AI is not only a security measure of data for an individual but also very critical to ensure trustworthiness in AI applications. The users share extensive data ranging from location to browsing history, biometric information, and much more using AI-powered tools, which are integrated into personal and professional life. In the absence of robust data privacy protocols, it could inadvertently expose personal information to some misuse and, consequently cause some highly adverse effects such as identity theft or other profiling not authorized and reputational damage. It is thus vital for data privacy in AI systems to instill an environment with safe innovation and user trust.
Challenges of Artificial Intelligence in Ensuring Data Privacy:
This brings along several challenges to the privacy of data as AI depends on wide datasets, often heterogeneous and containing highly sensitive information. Some key issues related to privacy in these areas are:
- Data Breaches: AI systems can become vulnerable to data breaches. It would easily cause leakage of such important personal data.
- Unauthorized Access: Without correct measures or controls, AI models may permit unauthorized parties to access personal data, which may lead to misuse.
- Privacy Inference Attacks: Some algorithms can indirectly infer the personal information, even though the latter themselves are not exposed explicitly.
- Data Retention Concerns: Certain AI systems retain data for too long, thus creating extended risks of privacy.
If one understands these challenges, one may develop policies and techniques to mitigate the related privacy risks without killing AI potential.
The Types of Data Collection for AI
AI systems use various forms of data, including:
- Personal Data: Names, addresses, contact information, and social identifiers.
- Sensitive Personal Data: health care information, biometric characteristics, financial information, geolocation data.
- Behavioral Data: Search history, social media interactions, app usage pattern, and online behaviors.
- Aggregated and Non-Personal Data: Information collected on groups or de-identified data that sometimes may be re-identifiable.
These data types feed AI models and, therefore, must be protected against misuse and unauthorized access.
Machine Learning and Data Security Risks
Such models, by extension, are exposed to various security risks. These are risks emanating from the vulnerability of how ML algorithms learn, process, and store data. Risks include
- Model Inversion Attacks: Attackers invert-engineer the training data, thereby exposing sensitive information.
- Membership Inference Attacks: the attacker can infer whether any given individual’s data belonged to the training set.
- Data Poisoning Attacks: Bad actors could inject corrupted data to the model, which alters the outcome and violates privacy.
This requires machine learning model protection from such threats, thereby furthering data privacy and prohibiting unauthorized exploitation of sensitive information.
AI and Data Privateness Regulation
Governments around the world have made stringent data privacy regulations following the boom in AI application. The said regulations enforce specific requirements concerning the collection, processing, and retaining of data, thus affecting the way any AI system handles data. Among the prominent regulations are:
- GDPR (General Data Protection Regulation): is a law related to data protection in the EU which mainly focuses on data collection requirements from the user with certain rights for access, correction, and deletion of one’s personal data.
- CCPA (California Consumer Privacy Act): Advocates for the rights of California residents pertaining to their personal information business holds concerning them.
- HIPAA (Health Insurance Portability and Accountability Act): Governs data privacy in healthcare, ensuring that health information used by AI applications in healthcare remains secure.
By following these rules, the data can be trusted and may avoid the infringement and reputational loss to organizations.
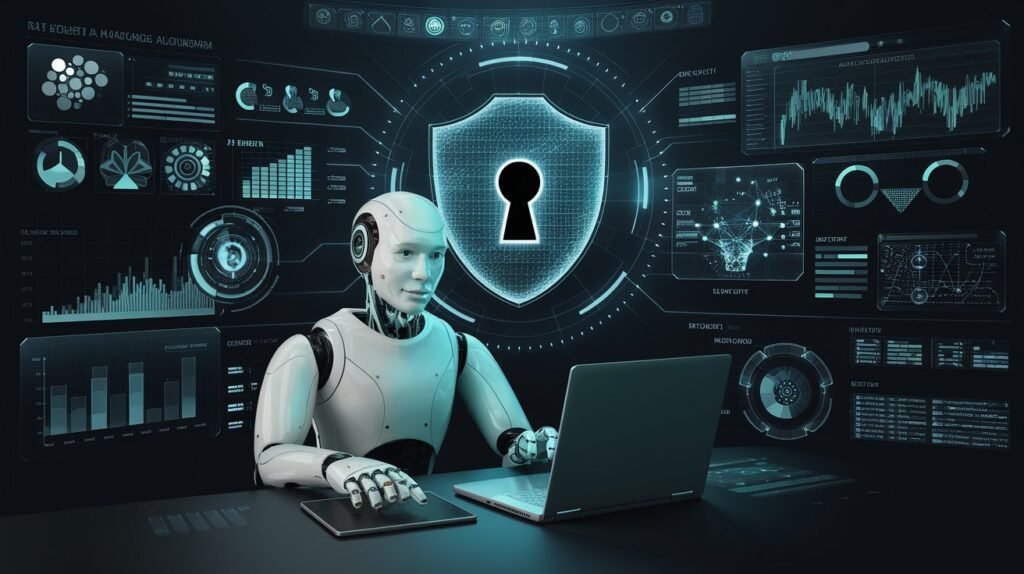
Privacy-Preserving AI Techniques
To ensure data privacy in AI applications, several privacy-preserving techniques have been developed. These enable the use of data without compromising user privacy, helping organizations balance data security and the need for robust AI models.
Federated Learning
Federated learning allowed the AI models to learn without transferability. Their knowledge is gathered without transferring the data to a central server. Data will remain on users’ devices while the updates of the model are forwarded to the central server. That way, it reduces the risk of breach without delivering extremely accurate models.
Differential Privacy
Differential privacy helps ensure that the output of an AI model does not reveal user individual data. This technique adds noise to data or to outcomes of models while still protecting privacy without compromising much with the quality of the model. In large datasets, differential privacy is extremely useful when individual data points have to remain confidential.
Homomorphic Encryption
Homomorphic encryption enables computations on encrypted data, allowing AI models to process sensitive information without decrypting it. This provides a powerful way to perform data analysis securely, especially for applications in finance and healthcare where data privacy is paramount.
Zero-Knowledge Proofs
It represents the manner in which zero-knowledge proofs validate data or knowledge without revealing that data. Zero-knowledge proofs, therefore, validate identity or other attributes without exposing the underlying data, which becomes high desirable in many applications involving privacy concerns.
Data Anonymization in AI
Data anonymisation removes any identifiable information, so a cloak of privacy remains, which prevents re-identification. Since anonymization is not perfect to prevent the person, advanced attackers may still be able to re-identify data, so such applications need additional privacy measures.
Synthetic Data
Synthesis Another privacy-friendly alternative is synthetic data, namely artificial datasets that mimic the statistical properties of real data, providing high-quality training data for AI models without risking leakage of sensitive information from the real world.
AI and the ethics of data privacy
Then there are AI-related ethical concerns that need to be met so that an ethical digital space that is safe and trustworthy is provided. Amongst which, one case would include ethical use of individual information, collected, used, and shared without full intent from the source. In AI systems, that predict behavior, detect patterns, and offer personalized services, risks infringement on people’s rights to privacy.
This would make ethics in organizations through the frameworks of AI, which will be on transparency, fairness, and accountability to make AI ethical. For instance, AIs should not serve to uphold bias or discrimination based on race, gender, or economic status. Organizations should disclose the use of data, create ways of improving control over information, and include methods that ensure care and protection for sensitive information.
Transparency in AI Models
Therefore, the transparency of AI models has an important role in trust and understandability. When the users know what is happening to their data, what decisions AI is making, and why it is making such decisions, then they will expand their trust towards the technology. The transparent AI model allows users questions and understanding of AI systems’ outputs while ensuring that data privacy and security concerns are discussed openly.
Explainable AI refers to a new and emerging area of aiming to design transparent models that can be explained to explain their decision-making processes. With XAI, organisations are able to give insights into how an AI model comes to its conclusions, thus augmented user trust and making it easier to spot biases or the potential misuses of the data. In many sectors, such as health care, finance, and law enforcement, the explanations from AI decisions will impact the daily lives of people.
Building Trust through Explainable AI
Explainable AI or XAI is what would most easily facilitate user trust in AI. This is because explainable AI has the potential of explaining how decisions are being made while the bounds of ethics and law are obeyed. Some of these questions can be answered by applying the concept of explainable models:
- Why did the AI make this decision?
- How does the AI really process my data?
- Can this model of AI be biased?
Answering all such questions, XAI equips users and stakeholders to hold the AI systems accountable; thus, it forms the foundation of trust and transparency indistinguishable with the responsible adoption of AI.
Bias and Fairness in AI and Privacy
Bias in AI is an issue that directly comes into play with data privacy and user trust. Any biased datasets on which AI models are trained have the potential to continue discriminatory practices, indicating how important fairness and bias detection can be to sustaining privacy and ethical standards. For instance, if an AI system for hiring or lending was based on biased data, it may not treat all groups fairly, thus violating privacy and ethics guidelines.
One of the ways that bias in an organization can be limited is through the presence of fairness auditing processes that ensure datasets have balanced data. Data privacy is intrinsic to this process, as it means that personal data is used equitably and respects the rights of all users. As such, addressing bias in AI not only protects data privacy but reinforces social equity and trust in AI systems.
Potential privacy risks from facial recognition AI
Another problematic application of AI is facial recognition technology, bringing with it big privacy concerns. On the one hand, this will enhance security and convenience. But it simultaneously opens a door for an unhealthy practice of misuse, such as surveillance, profiling, and identity theft.
Facial recognition tends to collect, store, and analyze face information sans consent from the individuals being monitored. This is a violation of the right to privacy in public areas since most people do not have an idea that they are being monitored. A number of countries and states have banned its usage, especially by forces of the law, to safeguard their rights to privacy.
This would require strict privacy protocols, including anonymity and/or encryption of facial data as well as explicit user consent prior to collection. In addition, proper policies for data retention, sharing, and deletion must be observed by organizations while being transparent about efforts to protect users’ privacy in facial recognition applications.
AI-based Privacy Policies
Improved privacy is another proactive approach of AI, which supports automatic privacy policy management. AIPrivacy rules are designed to change in real-time according to the changing behavior and preferences of its user, thus giving data privacy a personalized solution. For example, AI may detect sensitive data in a system and automatically apply rights on privacy or even warn users about the possibility of being exposed to their privacy.
AI-driven privacy policies use natural language processing, which can make the privacy terms and conditions much simpler to understand the treatment of users’ data. Automation of privacy management may assist in making it a more transparent and user-friendly proposition for data privacy, putting users in control of their data.
Third Party Data Sharing and AI Privacy
Indeed, data sharing is very prevalent in AI development where companies could buy or sell a dataset to correct model accuracy. That creates a problem in the sphere of privacy since data is exposed without permission and one loses control over how data is being used. When data has been transmitted to third parties, tracking and protection prove laborious processes prone to violating privacy.
Organizations can also reduce these risks with strict data-sharing agreements, third-party vendor due diligence, and having protocols in place for handling data. Further, the risk of privacy threats in data sharing across organizations can also be reduced through the techniques of anonymization, thus providing protection to sensitive data during the process of data sharing.
To secure AI systems against cyber threats.
Cyber criminals take advantage of a security hole to achieve unauthorized access to tons of sensitive information processed by these AI systems. Since the confidential data are put on the line by means of data breaches, hacking, and malware attacks, cyber threats likely compromise users’ privacy.
To counter these new threats, the organizations should plan with stern defense mechanisms such as encryption and firewall, along with multi-factor authentication. In addition, sustained vulnerability assessment identifies vulnerabilities of AI systems and patches them to reduce the possible intrusion threat. Therefore, protection of AI systems from cyber attacks safeguards data privacy and ensures proper use and security in application of AI technology.
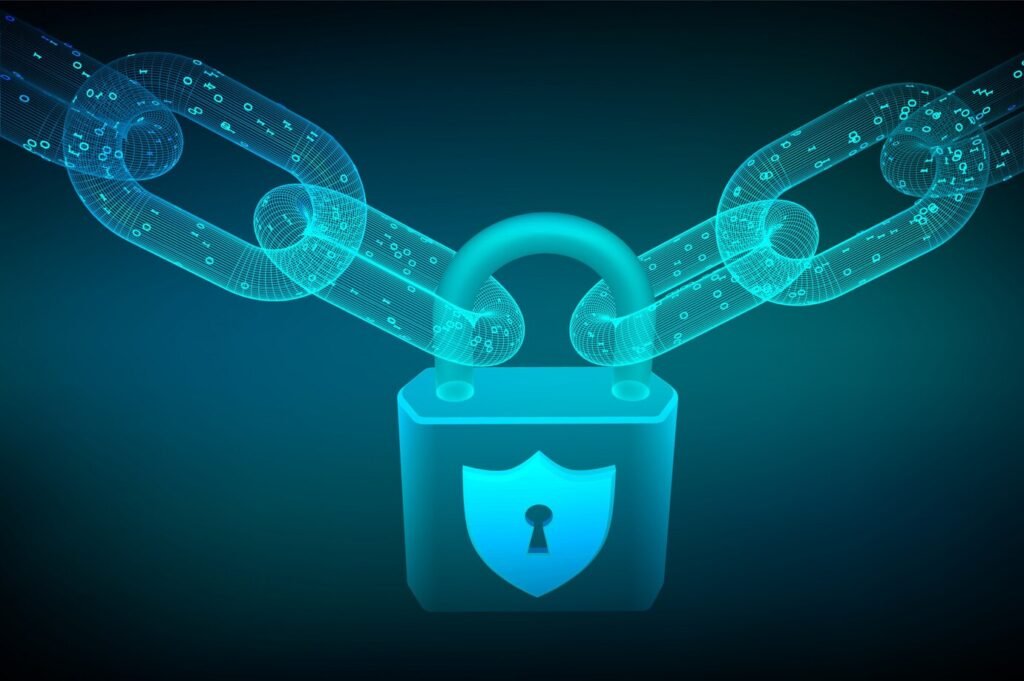
Data Governance and AI Privacy
Data governance is one of the AI privacy components; it simply refers to a framework responsible for data asset management and hence on how data information is obtained, utilized, stored, and deleted, thereby indicating personal data is to be treated based on the rules of privacy.
Strong data governance practices also entail regular auditing, data access controls, and user consent management. These provide the organizational capacity to control its data, thus preventing unauthorized access and minimizing risks arising from data breaches. Companies would balance their power in AI on the solid ground of a data governance framework by using the need for data privacy as a means of establishing a safe and ethical bedrock for AI applications.
User Control over Personal Data
One of the basic premises regarding data privacy in the AI age is empowering users to be in control of their own personal data. With this empowerment, people will have ownership over and trust their data and how this can be used, and organizations would have to give the following features to their users:
- Data Access: Users have the right to view and access collected data on them.
- Data portability: It enables users to move data between services.
- Data Erasure: Offering choices to the users for deletion and restrictions of any usage of their data.
In this regard, such measures help organizations align AI practices with expectations on privacy, so that one feels safe enough to input their data in an AI-driven environment.
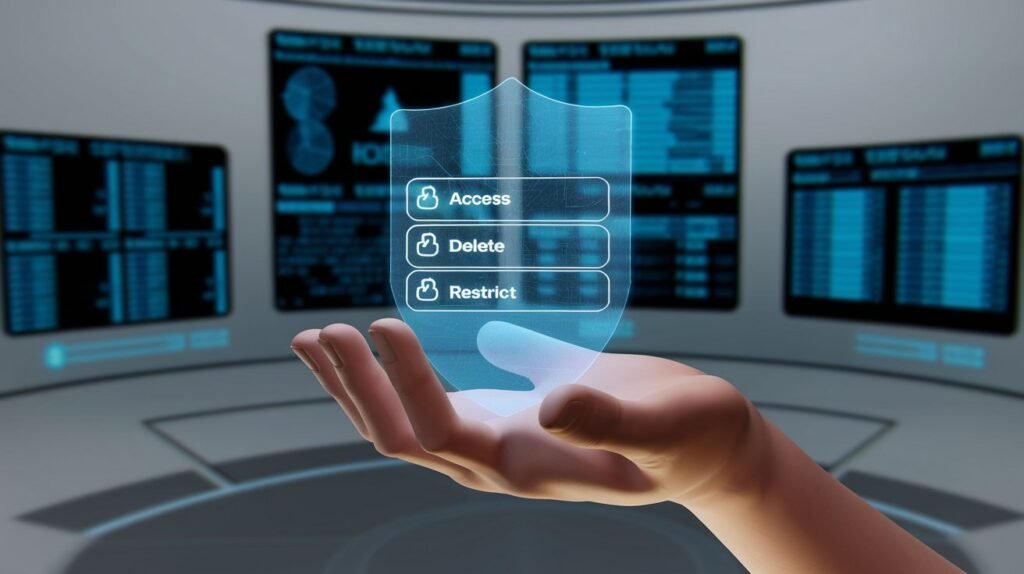
Future of AI and Data Privacy
The future of AI and data privacy will most likely develop with advanced technologies and corresponding adaptability in regulations. However, factors emerging in this trend include the growing use of privacy-enhancing technologies, decentralized AI that reduces data sharing, and advanced encryption to protect sensitive information. More hope lies in the expectation that regulatory bodies worldwide will introduce more comprehensive frameworks for ensuring data privacy hence formulating new standards for AI systems.
Data privacy will remain at the center of the ethanol production process when achieving ethical, trustworthy, and secure AI applications accelerates AI innovation. Organizations paying attention to data privacy in their AI strategy will conform not only to the standards of regulations but also boast of a competitive advantage through user trust and loyalty.
Conclusion
That is where artificial intelligence and data privacy interleave because, whereas personal information must be protected in an era when proper development of AI is desired, organizations can unlock the potential of AI and at the same time not lose privacy by embracing privacy-preserving techniques while complying with data protection regulations and prioritizing user trust. As AI continues to transform industries and society, there will be a need for balanced innovation and data privacy, which shall encourage the creation of safe, clear, and ethical digital environments for all.